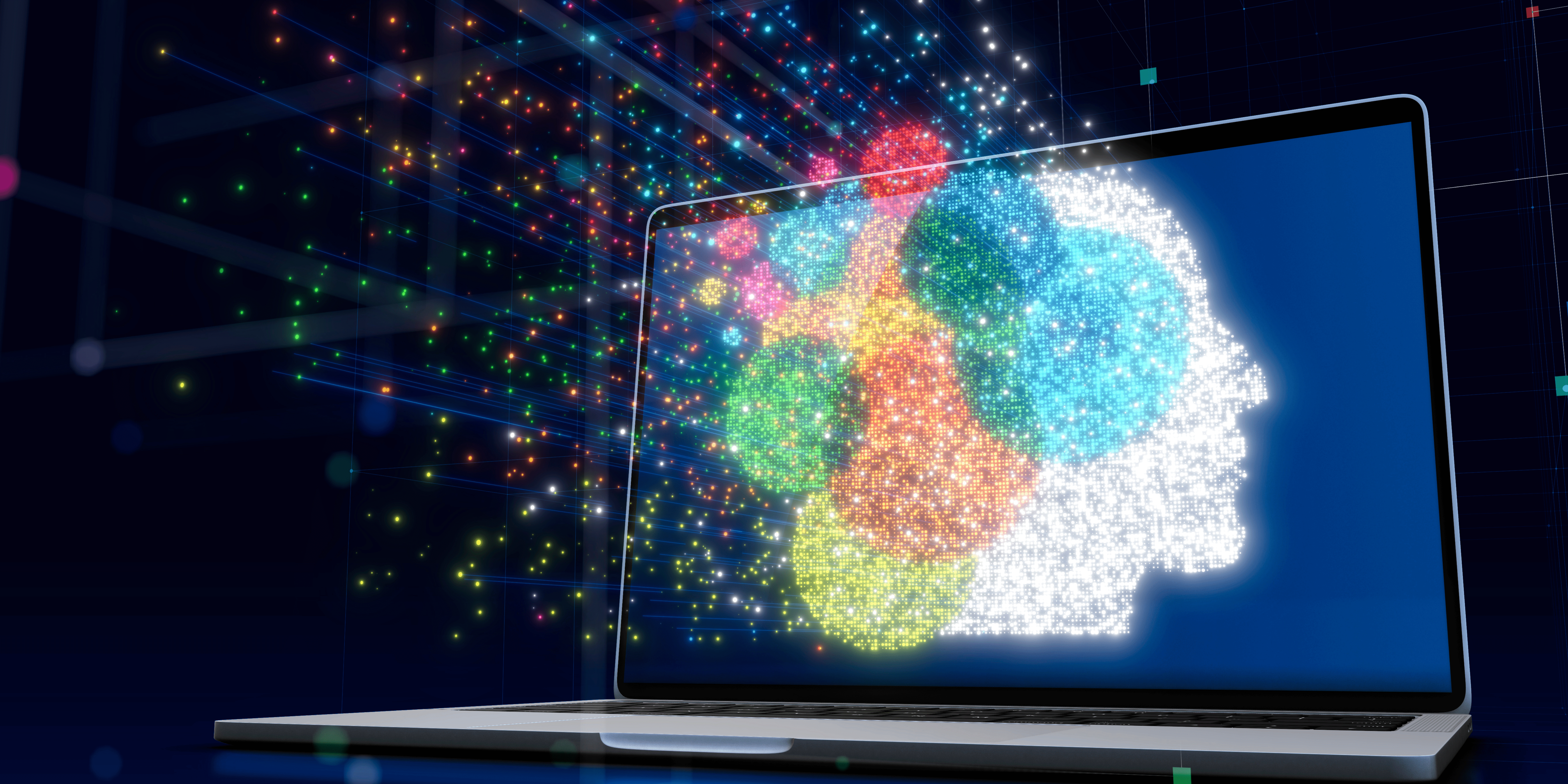
Beyond GANs: The Next Frontier in Generative AI Exploration
- Technology
- November 17, 2023
- No Comment
- 20
Introduction: Generative Adversarial Networks (GANs) have been a transformative force in the field of artificial intelligence, giving rise to incredible advancements in image generation, style transfer, and more. However, as technology marches forward, the landscape of Generative AI is evolving beyond GANs, ushering in a new era of innovation. In this blog, we will delve into the latest developments, emerging models, and the exciting possibilities that lie beyond GANs.
Understanding GANs and Their Impact:
Generative Adversarial Networks, introduced by Ian Goodfellow and his colleagues in 2014, have been instrumental in generating realistic data by pitting two neural networks, a generator, and a discriminator, against each other. GANs have found applications in image synthesis, artistic style transfer, and even text-to-image generation, marking a significant leap in AI capabilities.
The Limitations of GANs:
While GANs have showcased remarkable achievements, they are not without limitations. Challenges such as mode collapse, training instability, and the generation of artifacts still persist. This has paved the way for researchers and engineers to explore alternative approaches to overcome these hurdles and unlock new possibilities in Generative AI.
The Rise of Variational Autoencoders (VAEs):
One notable advancement is the increasing prominence of Variational Autoencoders (VAEs). Unlike GANs, VAEs operate with a probabilistic framework, emphasizing the generation of diverse outputs. This approach addresses some of the shortcomings of GANs, offering more stability in training and improved control over the generative process.
GPT and the Language of Creativity:
The rise of models like OpenAI’s GPT (Generative Pre-trained Transformer) has extended the scope of Generative AI beyond visual domains. GPT models are pre-trained on vast datasets, enabling them to generate coherent and contextually relevant text. This has sparked a revolution in natural language generation, with applications ranging from content creation to conversational AI.
Reinforcement Learning and Generative AI:
Reinforcement Learning (RL) is another avenue that holds great promise in the realm of Generative AI. By incorporating RL techniques, AI systems can learn to generate content through a process of trial and error, leading to more adaptive and context-aware generative models. This approach has shown potential in gaming, robotics, and dynamic content creation.
Adversarial Variational Bayes (AVB) and Hybrid Approaches:
Adversarial Variational Bayes (AVB) represents a hybrid approach that combines the strengths of GANs and VAEs. AVB aims to achieve the diversity of VAEs while maintaining the training stability associated with GANs. This fusion of methodologies showcases the dynamic nature of research in Generative AI, where interdisciplinary approaches lead to groundbreaking solutions.
The Emergence of Transformers in Image Generation:
Transformers, initially designed for natural language processing tasks, have made a significant impact on image generation. Models like DALL-E, developed by OpenAI, demonstrate the capabilities of transformers in creating diverse and highly imaginative images based on textual descriptions. This cross-domain applicability signifies a shift towards more versatile and universally applicable generative models.
Ethical Considerations and Responsible AI:
As Generative AI continues to advance, ethical considerations become paramount. Ensuring responsible use of generative models, preventing malicious use, and addressing issues related to bias and fairness are critical aspects of the evolving landscape. Researchers and practitioners are actively working towards establishing ethical guidelines to govern the development and deployment of Generative AI technologies.
Future Outlook:
The future of Generative AI is undeniably exciting. Continued research into novel architectures, improved training methodologies, and interdisciplinary collaborations are expected to push the boundaries further. From generating highly realistic virtual environments to aiding in drug discovery through molecular design, the applications of Generative AI are poised to revolutionize diverse industries.
Conclusion:
As we venture beyond GANs in the realm of Generative AI, the landscape is brimming with possibilities. From VAEs to transformers and hybrid approaches, researchers are paving the way for more stable, diverse, and context-aware generative models. The fusion of creativity and technology is reshaping how we perceive and interact with artificial intelligence, opening doors to a new era of innovation. The journey beyond GANs is not just a leap forward; it’s a testament to the boundless potential of Generative AI in shaping the future.